Maximizing Patient Care through AI-Enhanced HCC Code Discovery
By leveraging Medical LLMs from John Snow Labs, WVU Medicine identified and extracted relevant HCC codes from clinical notes. Newly found diagnosis codes are then communicated to clinicians for review directly inside the EHR.
-
Streamlined HCC coding
-
Enhanced accuracy and
completeness -
Improved patient outcomes
The Problem
Research consistently suggests that using only structured data, such as diagnosis codes from problem lists or claims, can mean missing or wrong codes for almost half of all patients
of Clinical Coding Using
ICD-10 for Ambulatory Visits
Volume 150, June 2021
The Solution
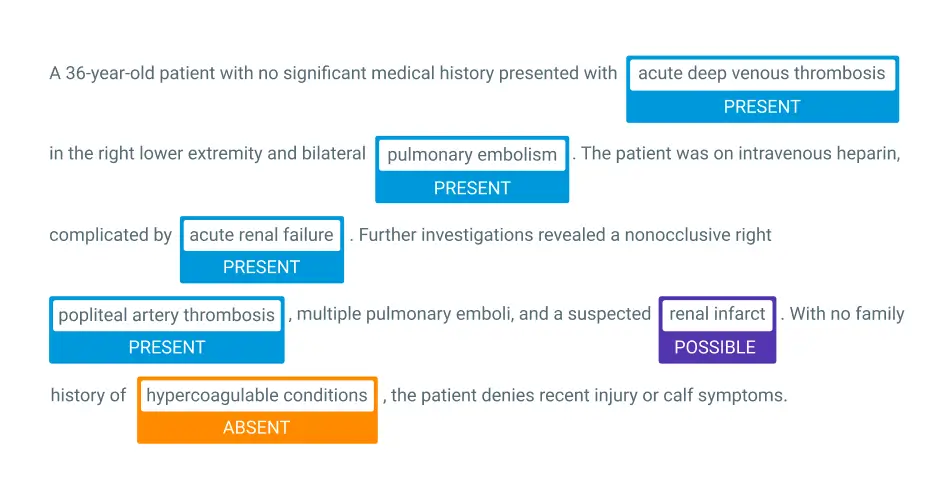
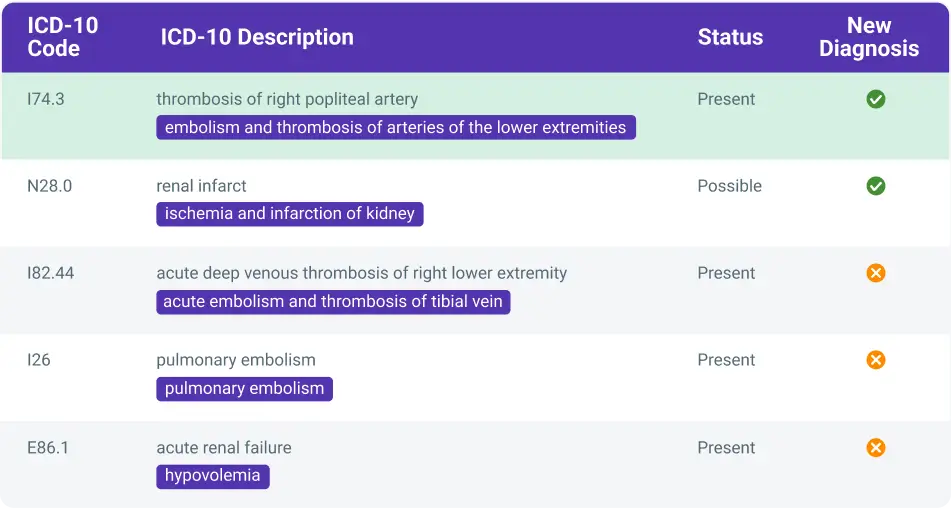
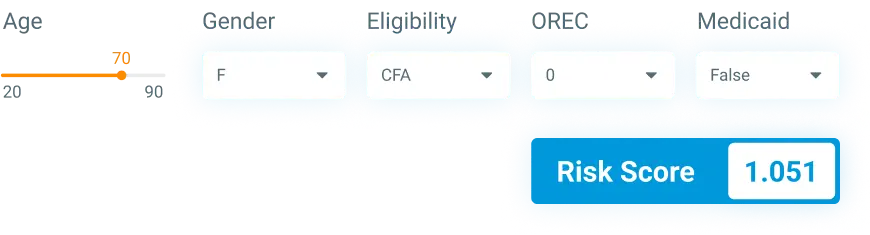
Automated integration into the EHR (for review by clinicians) or the Generative AI Lab (for review by clinical coders).
The user interface includes a full audit trail, work queues, team management, analytics, medical terminologies, and an API for automated integration into downstream workflows.
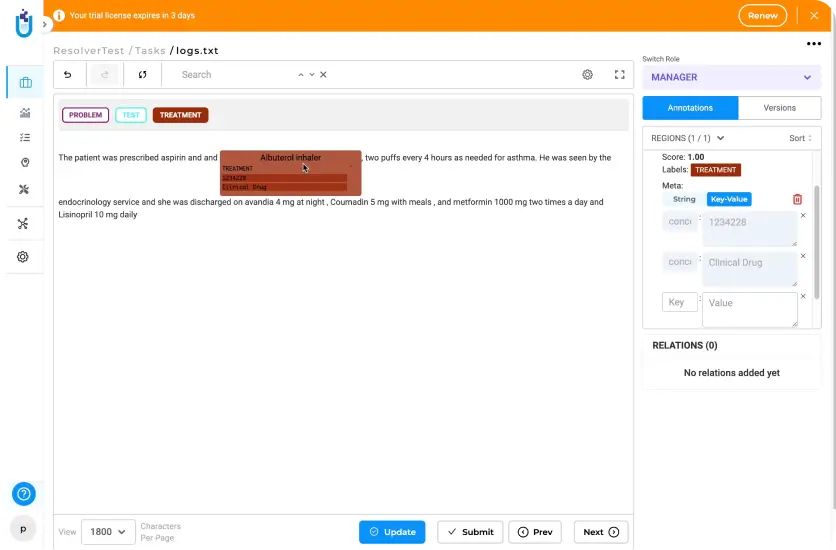
Automated Patient Risk Adjustment and Medicare HCC Coding from Clinical Notes
See how John Snow Labs and Databricks built a solution that derives missed ICD codes, preventing lower risk adjustment for patients and lost revenue for the provider.
The solution delivers a secure, enterprise-ready environment for payers or at-risk providers.