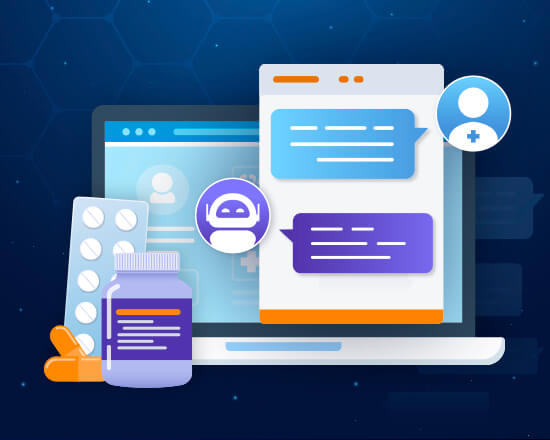
LLMs and Their Role in Telemedicine and Remote Care
Large Language Models (LLMs) are advanced artificial intelligence systems developed to understand and generate text in a human-like manner. LLMs, such as OpenAI’s GPT-4, have been trained on extensive datasets, allowing them to comprehend complex medical literature, clinical guidelines, and patient records. Their primary role in telemedicine and remote care is to enhance healthcare delivery by providing clinicians with accurate, data-driven insights and improving patient engagement through automated digital assistants. In this way, LLMs optimize diagnostic processes, refine virtual consultations, and improve the management of electronic health records (EHRs).
In telemedicine and remote care, where patient-provider interactions occur primarily through virtual platforms, LLMs act as criticaal intermediaries. LLMs can conduct initial patient assessments via automated interviews, guide patients through the triage process, and summarize key medical information for clinicians to prioritize urgent cases while ensuring every patient receives the information and care they need.
Furthermore, LLMs in telemedicine analyze patient histories, identify relevant symptoms, and provide clinicians with recommendations based on the latest medical research. Their ability to streamline routine tasks like symptom checking, follow-ups, and scheduling enables healthcare professionals to focus on complex cases. LLMs play a crucial role in managing comprehensive patient records, ensuring patients receive personalized and culturally sensitive care, regardless of location.
As telemedicine increasingly relies on LLMs and other digital health technologies to address patient demands and geographical barriers, LLMs are becoming critical. LLMs in telehealth can overcome language barriers, handle complex medical queries efficiently, and integrate with health systems to provide comprehensive virtual care. They help coordinate patient care by linking healthcare professionals and resources, creating a cohesive telemedicine ecosystem. LLMs’ ability to analyze and interpret medical data in multiple languages ensures diverse populations receive high-quality, tailored care. This role ultimately maximizes telemedicine’s global impact.
To summarize, LLMs in telemedicine and remote care are essential. They streamline patient assessment, provide intelligent triage and diagnostic support, and optimize clinical workflows. Leveraging their data-driven insights, healthcare providers can deliver accurate, efficient virtual care globally. LLMs are crucial in telemedicine and remote care.
Overview of the Healthcare Challenges LLMs Address in Telemedicine and Remote Care
The healthcare industry faces various challenges that Large Language Models (LLMs) are well-equipped to address, particularly in telemedicine and remote care. One significant issue is the shortage of healthcare professionals in underserved regions. While telemedicine helps bridge this gap, healthcare providers often struggle to handle the high volume of patient inquiries. LLMs alleviate this burden by automating tasks such as answering frequently asked questions and collecting initial patient information, freeing up doctors to focus on the most critical cases.
Another major challenge is managing the vast amount of data generated in healthcare. Providers must remain current on emerging research, clinical guidelines, and treatment approaches. LLMs offer invaluable assistance by summarizing medical studies and delivering evidence-based recommendations tailored to individual patients.
Managing Electronic Health Records (EHRs) can also be overwhelming. LLMs streamline this process by summarizing patient histories, identifying health trends, and highlighting crucial data for quick reference. This improves clinical workflows and ensures that patients receive accurate and timely care.
Language barriers present an additional challenge in healthcare delivery. LLMs can translate information to facilitate communication between providers and patients who speak different languages, ensuring that non-native speakers receive comprehensive care. By providing tailored and accurate translations, LLMs help reduce misunderstandings and foster trust between patients and clinicians.
Overall, LLMs in telemedicine and remote care play a pivotal role in improving healthcare delivery by streamlining information management, enhancing provider-patient communication, and allowing healthcare professionals to focus on what matters most.
Emerging Technologies Complementing LLMs
In the rapidly evolving healthcare landscape, several emerging technologies complement LLMs to create a comprehensive telemedicine ecosystem. Wearable devices and the Internet of Things (IoT) provide real-time patient data, enabling LLMs to analyze vitals and activity levels, alerting clinicians to potential health issues. For instance, data from smartwatches can be used to monitor heart rates and detect early signs of cardiovascular issues.
Machine learning algorithms and predictive analytics enhance the capabilities of LLMs by identifying patterns in patient data, offering predictive insights into disease progression. These models are crucial for chronic disease management and early detection of emerging health risks.
Robotic Process Automation (RPA) integrates seamlessly with LLMs to automate repetitive administrative tasks, such as scheduling appointments and processing insurance claims. This further reduces the administrative workload on healthcare providers, allowing them to focus on patient care.
Blockchain technology offers secure, decentralized data management, ensuring that patient data remains tamper-proof. By integrating blockchain with LLMs, telemedicine platforms can enhance data privacy and security compliance.
In summary, LLMs play a critical role in transforming telehealth and remote care by addressing the industry’s most pressing challenges. Their rapid analysis, understanding of medical language, and ability to automate processes complement emerging technologies to create an intelligent, patient-centric healthcare system.
Benefits of Using LLMs for Telemedicine and Remote Care
Enhanced Patient Interaction and Triage
Large Language Models (LLMs) significantly enhance patient interaction in telemedicine by streamlining communication and providing accurate information. They serve as virtual assistants, capable of answering common health questions, guiding patients through a triage process, and identifying cases that require immediate attention.
- Automated Triage: LLMs facilitate efficient patient screening by collecting essential information through chatbots or automated forms. By analyzing symptoms and medical histories, they categorize cases based on urgency and suggest initial steps before a healthcare provider’s involvement. This system allows clinicians to prioritize critical cases while ensuring all patients receive appropriate guidance.
- Efficient Communication: Virtual assistants provide immediate responses to frequently asked questions, reducing the need for patients to wait for a healthcare provider’s availability. They offer accurate medical advice on topics like medication adherence, lifestyle changes, and symptom management. This immediate access helps alleviate patients’ anxiety and empowers them to take better control of their health.
- Personalized Engagement: LLMs utilize patient data to tailor their interactions. For example, they might remind patients with diabetes to monitor their blood sugar levels or encourage cardiovascular patients to maintain a heart-healthy diet. This personalized engagement fosters trust and encourages patients to follow treatment plans, leading to improved health outcomes.
Diagnosis and Treatment Recommendations
LLMs provide healthcare providers with valuable support in diagnosing and treating various medical conditions. Their vast training on medical literature and clinical data allows them to generate accurate recommendations based on patient symptoms and historical information.
- Differential Diagnosis: With their deep understanding of medical knowledge, LLMs can analyze symptoms and suggest potential conditions that match a patient’s profile. For instance, when a patient presents with a persistent cough and fever, an LLM might propose diagnoses such as pneumonia, COVID-19, or tuberculosis and recommend further tests to confirm the specific condition.
- Treatment Plans: Once a diagnosis is made, LLMs suggest treatment plans that align with the latest clinical guidelines. They consider factors like patient age, allergies, and existing medical conditions to recommend medications, therapies, or lifestyle adjustments. These suggestions improve decision-making and ensure that patients receive personalized care.
- Remote Monitoring: For chronic conditions, LLMs analyze data from wearable devices and online consultations to monitor patients over time. They alert healthcare providers to changes that may indicate a worsening condition, prompting timely intervention.
Electronic Health Record (EHR) Management
Managing Electronic Health Records (EHRs) is crucial in healthcare but can be time-consuming for clinicians. LLMs automate and optimize many aspects of this process, improving data accuracy and accessibility.
- Automated Summaries: LLMs can analyze extensive patient records and summarize critical information, highlighting relevant medical history, allergies, and current medications. This summary allows clinicians to quickly understand the patient’s condition without sifting through pages of data.
- Identifying Health Trends: LLMs analyze historical data to detect long-term health trends that may indicate chronic conditions or emerging health risks. For instance, a gradual increase in blood pressure readings might signal hypertension, prompting early intervention.
- Accurate Documentation: Natural Language Processing (NLP) capabilities enable LLMs to convert spoken or written notes into structured EHR entries. This feature reduces the administrative burden on healthcare providers and minimizes the risk of errors in documentation.
- Data Accessibility: By organizing patient records effectively, LLMs make it easier for different healthcare providers to access relevant data. This accessibility is essential in multidisciplinary care, where specialists must collaborate and share information seamlessly.
Medical Education and Training
LLMs also play a vital role in medical education and training by offering up-to-date information and guiding clinicians through complex cases.
- Clinical Guidelines and Research Summaries: LLMs continuously analyze medical literature and clinical guidelines, summarizing findings into digestible insights for healthcare professionals. This information ensures that clinicians stay updated with the latest medical advancements and apply evidence-based practices.
- Case-Based Learning: When presented with patient data, LLMs can identify similar cases and illustrate how healthcare providers handled those scenarios. This case-based approach helps clinicians understand best practices and apply them in real-world situations.
- Medical Simulations: LLMs can simulate patient interactions and clinical scenarios, enabling trainees to practice decision-making skills in a safe, controlled environment. They receive immediate feedback, which helps refine their diagnostic and treatment approaches.
- Continuous Learning: By providing quick answers to clinical questions, LLMs support continuous learning for healthcare professionals. This ability allows them to handle unfamiliar conditions confidently and deliver high-quality care.
Language Translation and Overcoming Barriers
Language barriers can significantly hinder effective healthcare delivery, especially in multicultural societies where patients and healthcare providers may not share a common language. LLMs help bridge this gap by offering real-time translations and ensuring that patients receive comprehensive care.
- Real-Time Translation: LLMs can translate patient inquiries and clinician responses, facilitating seamless communication between non-native speakers and healthcare providers. This translation is particularly useful in telemedicine, where patients often provide detailed descriptions of their symptoms and medical histories.
- Cultural Sensitivity: Beyond translation, LLMs understand the cultural nuances that influence healthcare delivery. They can tailor their responses to address specific concerns that may arise from cultural beliefs or traditional practices.
- Medical Jargon Simplification: Healthcare information is often dense and filled with complex terminology. LLMs simplify medical jargon, explaining conditions and treatment plans in layman’s terms to ensure patients understand their health status and care instructions.
- Patient Empowerment: By breaking down communication barriers, LLMs empower patients to take a more active role in their healthcare. They can accurately convey their concerns, ask questions, and make informed decisions about their treatment.
In conclusion, LLMs offer transformative benefits in telemedicine and remote care, enhancing patient interaction, diagnosis, EHR management, education, and language translation. Their ability to analyze data rapidly and generate accurate, personalized insights makes them invaluable tools in addressing the challenges of modern healthcare. As these models continue to evolve, their integration into telemedicine platforms will further revolutionize healthcare delivery, ensuring accessible, efficient, and high-quality care for all patients.
Large Language Models in Telediagnosis of Clinical Conditions
Definition of Telediagnosis and Its Applications in Telemedicine
Telediagnosis, a cornerstone of telehealth, involves diagnosing medical conditions remotely using digital health technologies. It leverages telecommunication and electronic health tools to facilitate patient examinations and deliver accurate, timely diagnoses. By utilizing virtual consultations, patient self-reported data, and medical imaging analysis, telemedicine telediagnosis allows healthcare providers to offer care without requiring patients to visit physical facilities.
The importance of telediagnosis in telemedicine has risen significantly due to the COVID-19 pandemic and the increasing demand for remote healthcare. Patients in rural areas, people with mobility challenges, and those needing specialist consultations greatly benefit from telemedicine’s telediagnosis services. Large Language Models (LLMs) play a crucial role in enhancing this practice by processing massive data volumes, offering personalized insights, and providing differential diagnoses. Specific Clinical Scenarios Where LLMs Are Valuable in Telemedicine:
- General Practice Consultations: LLMs streamline triage processes in telemedicine by conducting initial patient interviews. They ask relevant questions about symptoms, medical history, and lifestyle factors, enabling primary care physicians to decide if patients require urgent care or specialist referrals.
- Chronic Disease Management: LLMs analyze patient-reported data, such as blood sugar levels or spirometry results, to detect trends indicating disease progression in patients with chronic conditions like diabetes or COPD. They offer tailored advice on lifestyle adjustments and medication adherence.
- Dermatology Consultations: In telemedicine, LLMs compare patient-uploaded images or symptom descriptions with extensive condition databases to provide preliminary assessments. They refer urgent cases to dermatologists for immediate attention.
- Mental Health Support: LLMs conduct mental health assessments in telemedicine, inquiring about mood, sleep patterns, and daily activities to identify depression and anxiety. They suggest coping strategies and guide patients to specialized mental health professionals.
- Pediatric and Geriatric Care: LLMs adapt their questioning to suit different age groups, understanding childhood illnesses and geriatric syndromes. They ensure early detection and timely intervention in both age groups.
In telemedicine, LLMs play a pivotal role in managing patient interviews, analyzing structured and unstructured data, and ensuring healthcare professionals provide comprehensive, accurate care. Their ability to process medical data quickly and offer insights makes them indispensable in enhancing healthcare delivery remotely. Analyzing Data, in Telemedicine and Remote Care Using Advanced Language Models:
- In the field of telemedicine, Advanced Language Models (ALMs) play a role in analyzing both unstructured data enabling healthcare professionals to offer precise and thorough diagnoses.
- Structured Data Analysis in Telemedicine; ALMs demonstrate proficiency in examining data such as vital signs, laboratory findings and patient demographics to identify patterns that may indicate specific medical conditions. For instance ALMs can detect trends in blood pressure readings that signal hypertension. By correlating symptoms with established conditions ALMs assist telemedicine practitioners in providing diagnoses for further evaluation by healthcare providers.
- Unstructured Data Interpretation in Telehealth; In the realm of telehealth and remote care unstructured data like patient records, clinician notes and medical imaging reports harbor insights. ALMs sift through these documents to extract details such as diagnoses or family medical histories that could impact the current health status. Additionally they decode terminology. Offer actionable guidance for healthcare professionals.
- Integration of Diverse Data Sources in Telemedicine; Through amalgamating information, from devices, laboratory tests and clinician observations ALMs construct profiles of patients receiving telehealth services. This holistic approach enables them to generate diagnoses that encompass aspects of an individuals health. For example Language Models (LLMs) can recognize signs, like tiredness, weight loss and stomach issues that may suggest someone has disease.
- In the field of telehealth LLMs consistently improve their ability to diagnose by incorporating data and staying up to date with telemedicine trends. This ongoing learning helps them adjust and enhance their skills offering precise insights for remote healthcare over time.
To sum up LLMs capacity to analyze both unstructured data is an asset in telehealth. This ensures that healthcare providers receive information, for prompt diagnoses. Supporting Radiology and Pathology Analysis with LLMs in Telemedicine and Remote Care:
- In telemedicine and remote care, radiology and pathology play a crucial role in confirming diagnoses. LLMs significantly support this process by analyzing medical images and histopathological data.
- Radiology Support in Telemedicine: LLMs assist radiologists by identifying abnormalities in X-rays, CT scans, and MRI images. They compare these medical images with extensive databases of known pathologies to flag areas of concern. For instance, an LLM can highlight potential nodules in a chest X-ray, suggesting further investigation into lung cancer—a critical feature in telemedicine where early detection is essential.
- Pathology Analysis: In pathology, LLMs analyze biopsy samples to identify cellular patterns indicative of diseases like cancer or autoimmune disorders. They help pathologists distinguish between benign and malignant growths and classify cancer stages based on cell morphology. This speeds up the diagnostic process and ensures consistency in pathology reports, particularly in telemedicine and remote care where accuracy is crucial.
- Natural Language Understanding in Radiology and Pathology Reports: LLMs interpret radiology and pathology reports, which often contain complex medical language. They translate these findings into actionable summaries that clinicians can easily understand, allowing for faster and more accurate decision-making in telemedicine.
- Reducing Diagnostic Errors in Telemedicine: Diagnostic errors in radiology and pathology can significantly affect patient care. LLMs reduce these errors by double-checking human assessments and offering alternative interpretations of the data. This additional analysis layer provides clinicians with a broader perspective and increases diagnostic confidence, enhancing telemedicine and remote care practices.
Overall, LLMs provide critical support to radiology and pathology in telehealth by improving the accuracy, speed, and reliability of diagnostic processes.
Addressing Challenges and Improving Accuracy with LLMs in Telemedicine and Remote Care
While Large Language Models (LLMs) offer significant benefits in telehealth, they face challenges in data privacy, clinical acceptance, and bias.
Data Privacy and Security: LLMs in telemedicine handle sensitive patient data, requiring secure transmission and storage to prevent breaches and maintain patient trust. Encryption and anonymization protocols are crucial to addressing these concerns, ensuring telemedicine practices remain secure and reliable.
Clinical Acceptance in Telemedicine: Some healthcare providers may hesitate to trust LLMs for diagnoses in telemedicine due to unfamiliarity with the technology. Building trust requires transparency in how these models generate insights and rigorous validation against clinical outcomes, so clinicians feel confident incorporating them into telemedicine and remote care.
Bias and Equity in Telemedicine: LLMs trained on biased datasets could unintentionally perpetuate existing healthcare disparities. Continuous monitoring and adjustment of training data are vital to minimize biases and ensure equitable care across diverse populations.
The Role of LLMs in Telemedicine and Remote Care: Despite these challenges, LLMs streamline patient interviews, analyze structured and unstructured data, and support radiology and pathology analysis in telemedicine. They empower clinicians to provide accurate, timely diagnoses while overcoming geographical barriers to healthcare access.
As these models evolve, their enhanced diagnostic accuracy will further bolster telemedicine and remote care practices, creating a more equitable, patient-centric healthcare system.
Trends of Large Language Models (LLMs) in Telehealth and Remote Care
The increasing popularity of using devices for monitoring in telemedicine and remote care
Wearable technology has now become a crucial element, in telemedicine and remote healthcare enabling medical professionals to keep track of patients continuously. These devices, which monitor signs, physical activity and sleep patterns produce an amount of data. This data can be analyzed by language models to offer health insights within the realm of telemedicine.
Continuous Health Monitoring; Smartwatches and fitness trackers collect real time information on heart rate blood pressure and oxygen levels. By analyzing this data advanced language models can identify trends. Recognize health issues such as heart rhythms or low oxygen levels. This ongoing monitoring is essential for managing conditions and allowing for interventions to prevent complications—an essential component of telemedicine.
Understanding Patient Behavior; Wearable devices keep track of activity levels and sleep habits providing information about an individuals lifestyle choices. Advanced language models examine these metrics in relation to health outcomes. Suggest lifestyle modifications for overall well being. For example if a wearable device detects decreased activity levels, an advanced language model might recommend increasing exercise to manage weight or improve health.
These insights play a role in the proactive care approach often seen in telemedicine. Automated Alert Systems; The integration of wearables, with language models leads to automated alerts being generated for both patients and healthcare providers. These notifications serve as signals of worsening conditions, in individuals with long term illnesses. Prompt them to adhere to their medications. For instance a spike in blood pressure might trigger an alert offering guidance to the patient and notifying the healthcare provider.
In the realm of Population Health Management, the amalgamation of data from devices allows LLMs to scrutinize patterns across communities. This approach facilitates healthcare providers in pinpointing trends identifying populations and distributing resources effectively thereby ensuring that telemedicine and remote care initiatives yield outcomes on a broader scale. The integration of wearables, with LLMs significantly boosts telemedicine by furnishing data driven insights that enhance monitoring, preventive care practices and the allocation of healthcare resources.
Healthcare IT Integration and System Interoperability in Telemedicine and Remote Care
Effective telemedicine and remote care rely heavily on seamless data sharing among various healthcare IT systems. LLMs play a critical role in fostering integration and interoperability, ensuring that information flows smoothly across platforms and stakeholders within the telemedicine ecosystem.
- EHR Integration: Electronic Health Records (EHRs) contain vast amounts of structured and unstructured patient data. LLMs can process this data, converting clinician notes and diagnostic reports into structured formats, making it easier for healthcare providers in telemedicine to access relevant patient information regardless of the original data format.
- Interoperability Standards: LLMs support interoperability standards like HL7 and FHIR, allowing diverse healthcare systems to exchange data efficiently. This standardization ensures that patient information remains accessible across multiple telehealth platforms and care providers.
- Data Normalization: Medical data is diverse, including lab results, imaging studies, and clinician notes. LLMs normalize this data by mapping and identifying relevant fields, ensuring consistent terminology and categorization across different systems. This reduces errors and enhances care coordination, crucial for telemedicine and remote care.
- Predictive Analytics: Integrating data from EHRs, wearables, and other sources, LLMs offer predictive insights that improve telemedicine care delivery. They can, for instance, predict patient readmission risks or identify individuals at high risk for chronic diseases based on their medical history and current health status.
Through improved integration, standardization, and predictive analytics, LLMs enhance interoperability and data sharing in telemedicine, creating more cohesive and accurate patient care pathways.
Intelligent Assistance in Clinical Decision-Making in Telemedicine and Remote Care
Large Language Models (LLMs) are becoming increasingly indispensable as intelligent assistants in clinical decision-making within telemedicine, providing evidence-based recommendations that help healthcare professionals deliver optimal care.
- Differential Diagnosis: LLMs analyze patient symptoms, medical history, and lab results to suggest potential diagnoses. They compare current cases with similar ones from their training data, offering clinicians a comprehensive list of conditions to consider. This support streamlines the diagnostic process and reduces the risk of missing conditions, improving telemedicine accuracy.
- Treatment Recommendations: After a diagnosis is made, LLMs suggest treatment plans that adhere to clinical guidelines and align with patient preferences. They can recommend medication dosages, therapy options, or lifestyle changes tailored to the patient’s unique health profile, helping telemedicine practitioners provide customized, high-quality care.
- Clinical Guidelines: LLMs are trained on the most recent clinical guidelines and research literature. They can rapidly retrieve and summarize this information, giving clinicians the latest insights on emerging treatment protocols. This ensures that telehealth professionals deliver evidence-based care and follow best practices.
- Second Opinions: In complex cases, LLMs offer valuable second opinions by cross-referencing patient data with global medical literature. This capability helps clinicians validate their assessments and explore alternative diagnostic or treatment strategies, leading to more comprehensive and confident decision-making in telemedicine.
With LLMs providing differential diagnosis, treatment recommendations, clinical guideline adherence, and second opinions, telemedicine practitioners can deliver accurate, patient-centric care that’s backed by cutting-edge data analysis. Future Advancements: Personalized Medicine and Population Health Analysis As advanced language models (LLMs) progress they are set to revolutionize telehealth and remote care by enhancing medicine and analyzing population health.
- Personalized Healthcare; The future of the field is moving towards customized treatments, for each patient. LLMs will examine information, lifestyle choices and medical backgrounds to pinpoint disease indicators and forecast how individuals will respond to treatments. This personalized approach will assist healthcare providers in tailoring therapies that reduce side effects and enhance patient outcomes.
- Pharmacogenomics; LLMs are poised to have an impact on pharmacogenomics the study of how genetic factors influence an individuals reaction to medications. By scrutinizing data alongside treatment results LLMs can pinpoint patients who may benefit from drugs or encounter adverse effects. This knowledge empowers healthcare professionals to select the medications for each patient.
- Analysis of Population Health; At a level LLMs will scrutinize data on population health to detect patterns and distribute resources effectively. They will evaluate determinants of health—like status and geographical location—to anticipate regions with elevated risks for conditions such, as diabetes or heart disease. This proactive strategy enables healthcare institutions to develop interventions.
- Predictive Healthcare Models; LLMs will enhance models that anticipate disease outbreaks, patient rehospitalizations and resource requirements.
These advancements will help healthcare providers get ready, for health risks and prevent overwhelming the system. Ethical and regulatory considerations are crucial as medicine and population health analysis progress. LLMs will play a role in ensuring data privacy and implementing compliance measures. In summary LLMs are transforming telehealth and remote care through trends, like patient monitoring integrating healthcare IT and making clinical decisions. Their capacity to analyze data forecast health results and provide advice will enhance healthcare delivery more. As these models progress they will introduce an era of medicine and population health management improving patient outcomes and reducing healthcare disparities.
Integration of LLMs into an Existing Telemedicine Business
LLMs in Telemedicine and Remote Care: Seamless Integration
The adoption of Large Language Models (LLMs) is revolutionizing telemedicine and remote care. LLMs enhance patient interactions, automate diagnoses, and personalize care. Their integration into existing telehealth businesses promises significant benefits but demands careful planning to navigate challenges. Challenges in Integrating LLMs:
- Technical Infrastructure: Integrating LLMs requires robust technical infrastructure, as LLMs demand high-performance servers to process large datasets in real time. Network latency can disrupt telehealth services reliant on rapid responses. Therefore, providers must establish high-speed, stable network connections, particularly in areas with limited connectivity.
- Data Management: LLMs rely on comprehensive, high-quality patient data for accurate insights. Managing this data from diverse sources, such as Electronic Health Records (EHRs), wearables, and imaging devices, requires standardization. Telemedicine businesses must ensure LLMs can access standardized data formats like FHIR to enhance interoperability and streamline the integration process.
- Data Security and Privacy: Managing sensitive patient data requires strict adherence to privacy laws like HIPAA (U.S.) and GDPR (Europe). Secure data transmission and storage are critical, particularly for cloud-based LLM deployment. Encryption, anonymization, and access controls are vital to ensure that LLMs in telehealth maintain data security and avoid unauthorized access.
- Cost and ROI: Implementing LLMs can be expensive due to investments in infrastructure, data acquisition, and talent. Telemedicine businesses should evaluate ROI by analyzing how LLMs improve clinical workflows, enhance patient outcomes, and reduce operational costs. Effective LLM deployment can boost patient satisfaction and optimize care delivery.
LLMs in telemedicine and remote care can revolutionize healthcare services by enabling intelligent assistance in clinical decision-making, automating diagnoses, and providing tailored patient care. Despite the challenges, careful integration of LLMs can lead to more efficient, patient-centric healthcare that leverages the full power of this technology.
LLMs in Telemedicine and Remote Care: Data Analysis and Interdisciplinary Cooperation
Integrating Large Language Models (LLMs) into telemedicine and remote care requires effective data analysis and interdisciplinary cooperation to maximize their potential. LLMs deliver robust diagnostic and therapeutic insights, but their successful implementation hinges on collaboration between data scientists, clinicians, and IT specialists. Collaborative Data Analysis for LLMs
- Interdisciplinary Collaboration: LLMs in telemedicine thrive when data scientists, clinicians, and IT specialists collaborate effectively. Data scientists are crucial for training and validating the models, ensuring that LLMs deliver accurate and meaningful insights. Clinicians provide essential domain expertise, ensuring that LLMs’ outputs remain clinically relevant. IT specialists manage data integration and security to ensure a seamless flow between systems, maintaining LLMs’ integrity and performance.
- Customized Models for LLMs: Tailoring LLMs to specific telehealth needs enhances their diagnostic relevance. For instance, a telemedicine service specializing in chronic disease management benefits from models trained on diabetes or hypertension datasets. Interdisciplinary teams align customized LLMs with clinical workflows to streamline diagnosis and treatment.
- Feedback Loops for Improvement: Continuous improvement is essential for LLMs in telehealth. Establishing robust feedback loops between LLM outputs and clinical outcomes ensures that models evolve based on real-world results. Clinicians’ input highlights alignment or deviation in predictions, which helps data scientists refine the models to enhance their diagnostic accuracy.
- Ethical Considerations: LLMs in telemedicine can inadvertently reflect biases in training data, exacerbating healthcare disparities. An interdisciplinary team is vital in monitoring and mitigating these biases, ensuring that LLMs provide equitable care for diverse populations. Maintaining ethical standards is crucial for LLMs to uphold patient trust and deliver fair, accurate care.
By prioritizing collaborative data analysis and interdisciplinary cooperation, LLMs in telemedicine and remote care can revolutionize the field. Their integration into telehealth businesses demands continuous refinement and ethical vigilance, ensuring these powerful tools deliver precise, patient-centric healthcare.
- LLMs in Telemedicine and Remote Care: Developing Accurate Remote Patient Monitoring
- Wearable Integration with LLMs: Large Language Models (LLMs) integrate seamlessly with wearable devices, providing real-time health insights crucial for telemedicine and remote care. By establishing APIs to feed wearable data directly into LLMs, these models can detect patterns and analyze trends in vital signs and activity levels. This integration allows LLMs to assist clinicians in monitoring chronic conditions and identifying early signs of disease progression.
- Predictive Algorithms in LLMs: LLMs use predictive algorithms to recognize patients at risk of hospitalization or disease flare-ups. They analyze historical health data and trends to generate timely alerts, helping healthcare providers intervene before complications arise. By understanding the patterns in a patient’s health journey, LLMs contribute to proactive and preventative care.
- Behavioral Monitoring with LLMs: Beyond physical metrics, LLMs analyze behavioral data such as sleep patterns and medication adherence. With these insights, clinicians can deliver tailored advice, supporting patients in maintaining healthy habits that align with their personalized treatment plans. This comprehensive monitoring fosters improved outcomes and patient engagement.
- Integration of LLMs with EHRs: Merging wearable data with Electronic Health Records (EHRs) creates a comprehensive overview of patient health. LLMs can identify and interpret correlations between wearable metrics and clinical outcomes, enhancing their predictive capabilities. This synergy allows LLMs to offer more accurate assessments, recommendations, and care management strategies in telemedicine.
- By harnessing LLMs in telemedicine and remote care, clinicians can establish a robust remote patient monitoring system that offers precise, proactive healthcare solutions.
LLMs in Telemedicine and Remote Care: Intelligent Diagnosis and Assistance Tools
- Virtual Assistants Powered by LLMs: Large Language Models (LLMs) play a crucial role in telemedicine platforms as virtual assistants. They conduct comprehensive patient interviews, collecting information about symptoms, medical history, and lifestyle factors to create detailed health profiles. This profile aids clinicians in guiding patient care effectively.
- Differential Diagnosis with LLMs: LLMs excel at analyzing patient data to suggest differential diagnoses, prioritizing conditions that need urgent attention. They compare current cases with historical data to provide evidence-based diagnostic suggestions, ensuring that clinicians have a broad view of potential health issues.
- Decision Support Tools via LLMs: Decision support tools, driven by LLMs, offer clinicians valuable treatment recommendations aligned with clinical guidelines and patient preferences. They suggest appropriate medication dosages, therapy options, and lifestyle modifications, tailoring these strategies to each patient’s unique health profile.
- Continuous Learning of LLMs: LLMs continuously learn from new patient data and clinician feedback, enhancing their diagnostic and assistance tools. This iterative learning allows them to refine recommendations, adapting to emerging clinical trends and insights for superior patient outcomes.
- Incorporating LLMs in telemedicine and remote care ensures intelligent, evidence-based diagnosis and assistance tools, empowering healthcare professionals to deliver accurate, personalized care.
Ensuring Security and Privacy Compliance of Large Language Models (LLMs), in Telehealth and Remote Healthcare
Upholding Security and Privacy Standards
The deployment of Language Models (LLMs) in telehealth necessitates a thorough focus on security and privacy. In light of the sensitivity and regulatory requirements surrounding information telehealth providers must adhere to established frameworks to guarantee that LLM systems safeguard privacy and uphold security.
Maintaining HIPAA Compliance with LLMs
Within the United States the Health Insurance Portability and Accountability Act (HIPAA) mandates measures for Protected Health Information (PHI). When integrating LLMs into telehealth platforms it is crucial to ensure that PHI remains securely stored, transmitted and processed—whether in physical formats.
Addressing GDPR Requirements for LLMs
In Europe the General Data Protection Regulation (GDPR) oversees data handling, including healthcare related information. LLM applications dealing with data must align with GDPR tenets such as consent management, data minimization practices and respecting individuals right to erasure.
Navigating Implications of the 21st Century Cures Act
This legislation guarantees patients access to their health records while enforcing standards for interoperability across healthcare IT systems. For LLM implementations enabling data exchange is paramount, alongside preserving patient confidentiality.
Additional Regulations, in Different Regions
Various areas, such as California with its Consumer Privacy Act (CCPA) have rules to follow. LLMs used in telemedicine need to be flexible enough to adhere to diverse privacy standards for compliance. Incorporating LLMs effectively into telemedicine services requires navigation of these frameworks giving priority to data encryption, anonymization and access control to address privacy and security concerns. Through strategizing LLMs can maintain the levels of security and confidentiality, in telemedicine and remote healthcare settings.
- Strategies for LLM Compliance in Telemedicine and Remote Care
- Comprehensive Risk Assessments: LLMs in telemedicine require regular risk assessments to identify vulnerabilities in data management. Providers must scrutinize the security of data both at rest and in transit, ensuring that any weak points in LLM systems are reinforced with stronger safeguards.
- Data Mapping for LLMs: Understanding data flow through telemedicine systems with LLMs is vital. Data mapping tracks patient data from its collection to storage and sharing, ensuring compliance at every step by adhering to the appropriate regulatory standards.
- Access Control: Implementing role-based access control for LLMs minimizes the risk of unauthorized data usage. By restricting access to specific personnel, only authorized staff can view certain patient data, reducing internal data leaks and safeguarding confidentiality.
- Training and Awareness: Effective compliance requires comprehensive training. Healthcare professionals, data scientists, and IT teams handling LLMs should understand data privacy importance, proper PHI management, and how to recognize emerging security threats.
- Third-Party Vendor Management: Telemedicine businesses often rely on third-party vendors for cloud storage and analytics of LLM-generated data. It’s crucial these vendors follow the same security standards and confidentiality protocols as the telemedicine providers themselves. Contracts must include clauses enforcing compliance with the required security frameworks.
- By following these strategies, telemedicine providers can ensure that their LLMs operate securely and comply with regulatory standards in telemedicine and remote care.
Data Security Measures, for Telemedicine and Remote Care Services
Ensuring the security of data is crucial for of telehealth platforms utilizing encryption methods. Techniques like the Advanced Encryption Standard (AES) are utilized to safeguard information during transmission and storage maintaining privacy during interactions and operations.
Protecting Patient Privacy
Techniques such as anonymization are employed to safeguard privacy by removing identifiable information from datasets used in telemedicine. This allows healthcare professionals and researchers to analyze data securely minimizing risks of identification through methods like pseudonymization and data masking.
Importance of Secure Cloud Storage
Cloud storage is vital for telemedicine and remote care services dealing with datasets required for effective operations. Providers must implement measures such as factor authentication, encryption and redundancy in their cloud environments. Regular audits of cloud services help. Address vulnerabilities, ensuring robust security.
Significance of Audit Logs
Maintaining detailed audit logs is essential for telemedicine platforms to track access to data accurately. These logs record who accessed data the type of information retrieved and the purpose behind it. Audit trails aid, in compliance efforts monitor data usage practices. Assist in identifying security breaches to uphold transparency and trust.
- Privacy Challenges with Training LLMs
- Large Datasets: Telemedicine and remote care rely on Large Language Models (LLMs) trained on extensive datasets, often containing sensitive patient information. Handling these data while safeguarding privacy is a challenge due to the volume and diversity of healthcare data needed to provide effective telehealth services.
- De-Identification Risks: Even anonymized patient data used in telemedicine can sometimes be re-identified if combined with other datasets. This necessitates extra protective measures like differential privacy, which introduces noise to the data to thwart re-identification attempts.
- Bias and Fairness: Healthcare datasets used for training LLMs may contain biases that impact predictions in telemedicine and remote care. For example, underrepresentation of certain demographics can lead to skewed outputs. To address this, careful data analysis and regular retraining are essential to ensure equitable care.
- Data Ownership and Consent: Patients must give informed consent for their data to be used in LLM training for telehealth. This requires transparent communication about data use, while also providing mechanisms for patients to withdraw their data if desired.
- Continuous Monitoring and Improvement: The evolving nature of LLMs demands continuous monitoring and auditing of their outputs in telemedicine. Regular assessments help identify potential privacy concerns and ensure compliance with data protection standards, while also improving the models for better patient care
Conclusion
The integration of Large Language Models (LLMs) into telemedicine is transforming the healthcare industry. By leveraging the vast computational power of these models, telehealth platforms can offer intelligent and highly personalized patient care. However, this integration requires addressing significant challenges in infrastructure, data management, interdisciplinary cooperation, and compliance. Here, we summarize the key findings and present a vision for the future of telemedicine, balancing innovation with security. Recap of Key Findings and Recommendations:
- Challenges in Infrastructure and Data Management: Integrating LLMs into telemedicine requires a robust technical infrastructure. Organizations must secure high-performance servers, ensure data interoperability, and comply with privacy regulations. Continuous risk assessments, staff training, and vendor management are essential to safeguard patient data.
- Data Analysis and Interdisciplinary Cooperation: Successful deployment of LLMs relies on collaborative efforts between data scientists, IT specialists, and clinicians. Data scientists refine the models, clinicians ensure clinical relevance, and IT teams secure data flow. Feedback loops between clinicians and data scientists help refine the models continuously.
- Remote Patient Monitoring and Intelligent Assistance: Wearables and predictive algorithms enable continuous monitoring of patient health. LLMs process this data to provide personalized health insights, detect early signs of disease progression, and guide clinicians through intelligent decision support systems.
- Security and Confidentiality Compliance: With healthcare data being highly sensitive, telemedicine platforms must implement strong encryption, anonymization, and cloud security measures. Privacy frameworks like HIPAA and GDPR require continuous monitoring to identify vulnerabilities and ensure compliance. De-identification risks, data biases, and patient consent remain crucial challenges.
Transformative Potential of LLMs in Telemedicine and Remote Care:
- Personalized Medicine: Large Language Models (LLMs) are key to personalizing telemedicine and remote care. By analyzing genetic data, lifestyle choices, and medical histories, they provide tailored treatment recommendations that minimize side effects and improve patient outcomes. They can also identify early signs of flare-ups in chronic diseases and suggest timely interventions based on clinical guidelines.
- Enhanced Patient Interaction: Virtual assistants powered by LLMs enhance telehealth by conducting initial patient interviews and assessments. These intelligent assistants engage patients with round-the-clock support and personalized health advice, helping them navigate their care journey more effectively.
- Clinical Decision Support: LLMs bolster clinical decision-making in telemedicine by suggesting diagnoses and treatment options based on evidence and historical data. They provide valuable second opinions, ensure clinicians adhere to best practices, and continuously expand their knowledge base with the latest medical research.
- Predictive Healthcare Models: Predictive models built using LLMs can forecast disease outbreaks, predict patient readmissions, and assess health resource needs. These insights empower telehealth organizations to better prepare for emerging challenges and manage their resources more efficiently, ultimately improving patient outcomes and reducing healthcare costs.
The Future of Telemedicine and Remote Care: Innovation vs. Security
Ethical AI Implementation: The integration of Large Language Models (LLMs) into telemedicine requires careful ethical considerations. To ensure equitable care, these models must be free of biases that can impact patient treatment. Transparent development and interdisciplinary collaboration will help mitigate biases and improve fairness in remote healthcare.
Continuous Security Improvements: With privacy regulations constantly evolving, telemedicine providers must dynamically update their compliance strategies. Enhanced encryption, data anonymization, and secure cloud storage practices will be essential to counter emerging cybersecurity threats, ensuring patient data remains protected.
Public Trust and Engagement: Building trust with patients is crucial in telemedicine. Clear consent processes and educating patients on how their data will be used are key to fostering trust and engagement. Offering opt-out opportunities further reassures patients, creating a transparent relationship between them and their care providers.
Scalable Infrastructure: Future telemedicine platforms need robust infrastructure to accommodate growing data demands. Investments in high-speed networks, AI analytics, and secure cloud storage will ensure scalable, reliable, and secure telehealth services.
Global Healthcare Equity: Telemedicine, empowered by LLMs, has the potential to democratize access to healthcare by extending quality services to underserved regions. By providing local clinicians with advanced medical insights and diagnostics, these tools can significantly reduce global healthcare disparities.
In conclusion, telemedicine and remote care’s future lies in the effective balance of innovation and security. By prioritizing data security, ethical implementation, and interdisciplinary collaboration, telehealth can become a transformative force in global healthcare, enhancing outcomes while reducing disparities in the years to come.