John Snow Labs is the De-facto Industry Leader for Medical Large Language Models.
State of the Art Medical Language Models
John Snow Labs’ commitment is to always provide you with state-of-the-art accuracy. Making that happen means that we:
Train and fine-tune dozens of models per week
Build a growing set of healthcare-specific LLM benchmarks
Develop and run automated tests for compliance and Responsible AI
Benchmark against every new LLM that anyone publishes
Fine-tune with proprietary data, annotated by medical doctors
Work with hardware and cloud providers to optimize LLM speed, size, and cost
Benchmark | John Snow Labs | GPT-4o | Med-PaLM-2 |
---|---|---|---|
Clinical Knowledge | 89.4 | 86.0 | 88.3 |
Clinical Assessment | 75.5 | 69.5 | 71.3 |
Medical Research Q&A | 79.4 | 75.2 | 79.2 |
Medical Genetics | 95.0 | 91.0 | 90.0 |
Anatomy | 85.2 | 80.0 | 77.8 |
Professional Medicine | 94.9 | 93.0 | 95.2 |
Life Science | 93.8 | 95.1 | 94.4 |
Core Concepts | 83.2 | 76.9 | 80.9 |
Clinical Case Analysis | 79.8 | 78.9 | 79.7 |
Average Score | 86.2 | 82.9 | 84.1 |
Accuracy on OpenMed Benchmarks |
Preferred in a Blind Evaluation by Medical Practitioners
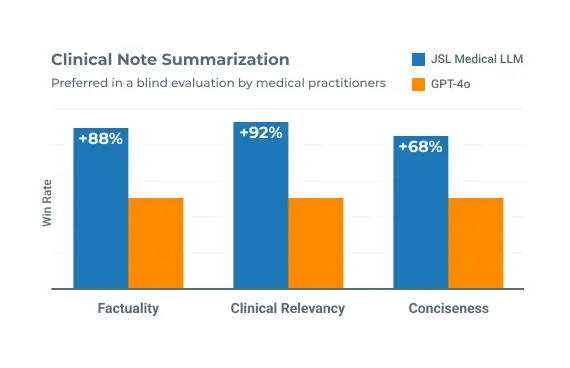
Clinical Note Summarization
Preferred 88% more often on factuality, 92% more often on relevance, 68% more often on conciseness compared to GPT-4o.
Sample Questions:
- Summarize the final pathological diagnosis of the lesion and the patient’s follow-up and recovery after surgery.
- Summarize the patient’s medical history and initial presentation.
- Summarize the background and objectives of the study from the given text.
Clinical Information Extraction
Preferred 46% more often on factuality, 50% more often on relevance and 45% more often on conciseness compared to GPT-4o.
Sample Questions:
- Can the TyG index be used to predict gestational diabetes mellitus (GDM) according to the following text?
- Given the note, what procedures did the patient undergo?
- Given the medical text, did Anlotinib benefit the patient?
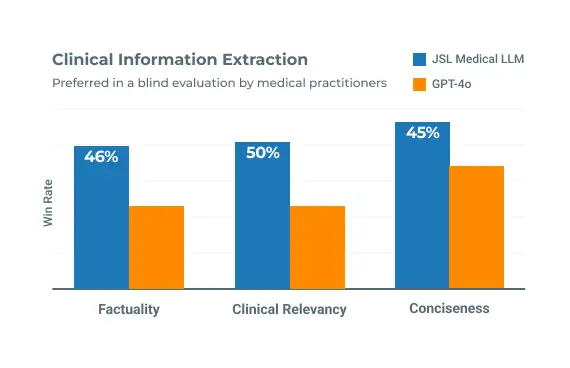
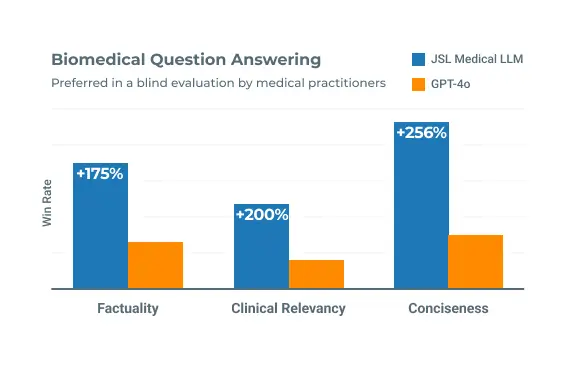
Biomedical Question Answering
Preferred 175% more often on factuality, 200% more often on relevance, 256% more often on conciseness compared to GPT-4o.
Sample Questions:
- Given the report, what biomarkers are commonly negative in APL cases?
- Given the note, why is the chemotherapy the mainly used treatment in TNBC patients?
- Given the article, what is sNFL used for?
Private and Compliance Deployment Within Your Network
Runs Privately
Deploy the Medical LLMs within your secure infrastructure, ensuring data sovereignty and full control over sensitive information.
No Data Sharing
Medical LLMs process data locally. No need for external data sharing or internet dependencies.
Built for Compliance
In line with privacy standards like HIPAA or GDPR, ensuring seamless integration into highly regulated environments.
Putting Healthcare LLMs to Production Use
Using Healthcare-Specific LLM’s for Data Discovery from Patient Notes & Stories
The US Department of Veterans Affairs, a health system which serves over 9 million veterans and their families. This collaboration with VA National Artificial Intelligence Institute (NAII), VA Innovations Unit (VAIU) and Office of Information Technology (OI&T) show that while out-of-the-box accuracy of current LLM’s on clinical notes is unacceptable, it can be significantly improved with pre-processing, for example by using John Snow Labs’ clinical text summarization models prior to feeding that as content to the LLM generative AI output.
Text-Prompted Patient Cohort Retrieval: Leveraging Healthcare LLM Models for Precision Population Health Management
Using John Snow Lab’s Healthcare LLM models, the ClosedLoop platform enables users to retrieve cohorts using free-text prompts. Examples include: “Which patients are in the top 5% of risk for an unplanned admission and have chronic kidney disease of stage 3 or higher?” or “Which patients are in the top 5% risk for an admission, older than 72, and have not undergone an annual wellness checkup?”
Applying Healthcare-Specific LLMs to Build Oncology Patient Timelines and Recommend Clinical Guidelines
This talk covers how applying healthcare-specific Large Language Models (LLMs) to Electronic Health Records (EHRs) presents a promising approach to constructing detailed oncology patient timelines. It explores how John Snow Labs’ healthcare-specific Large Language Model (LLM) offers a transformative approach to matching patients with the National Comprehensive Cancer Network (NCCN) clinical guidelines. By analyzing comprehensive patient data, including genetic, epigenetic, and phenotypic information, the LLM accurately aligns individual patient profiles with the most relevant clinical guidelines. This innovation enhances precision in oncology care by ensuring that each patient receives tailored treatment recommendations based on the latest NCCN guidelines.
Lots of companies make claims about healthcare-specific LLM’s. John Snow Labs are the only ones who publish reproducible accuracy benchmarks and have Medical LLM systems in production.