The emergence of data science and big data can add more power to the field of system dynamics as it will extend its applications. At the same time, system dynamics models can illustrate more interpretation for big data through its causal structure. In systems language we can call this a “Success to The Successful Archetype”.
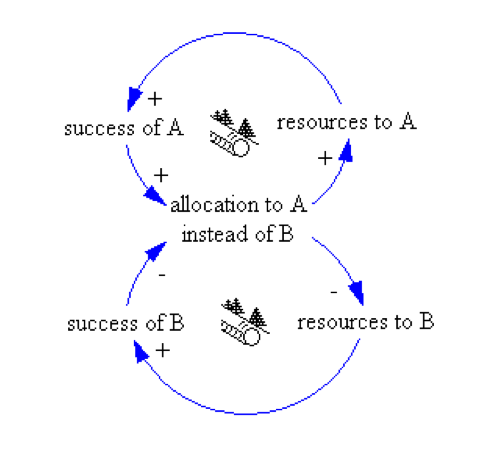
A success to the successful archetype
Let us understand together what does this mean and what can be the future of system dynamics in the scope of the great progress in the field of data science.
What is Systems Thinking?
It is the art and science of illustrating and describing inter-relationships between the system and its underlying parts and structures depending on causalities methodologies. In other words, it is a deeper understanding of the behavior of the system. People can be divided into two groups based on their overall view for the world around them. They can either be event-oriented thinkers or system thinkers.
What is System Dynamics?
It is more concerned with the quantitative and qualitative analysis for the impact of the interrelationship between complex systems and their underlying parts using computer-aided methodologies. It is more towards testing hypothesis.
The Systems Archetypes
Scientists have agreed on some common behavioral patterns according to their experience with different organizations. Those repeated behavioral patterns can tell us the future consequences taking into consideration the time factor and incidents (events) as two variables.
There are ten famous common archetypes:
- Limits to Growth
- Shifting the Burden
- Eroding Goals
- Escalation
- Success to the Successful
- Tragedy of the Commons
- Fixes that Fail
- Growth and Under-investment
- Accidental Adversaries
- Attractiveness Principle
System Dynamics Road Maps
System Dynamics was born in Sloan School of Management Massachusetts Institute of Technology (MIT) by Professor Jay W. Forrester in the 1950’s.
System Dynamics Road Maps is a series of self-study guide for learning and understanding system dynamics. The whole Road Maps are available for free download from MIT at:
How can SD models be useful?
They can help in prediction (note how predictive analytics achieved a great progress in the last years since the emergence of data science). This can be a clear evidence on the role both big data and system dynamics can play together.
System dynamics (SD) models can also illustrate the consequences of certain policy choices. It can be considered an aid for decision-makers as they can predict the results and effects of their decisions and choices in the future through SD models.
Moreover, SD models can explain the narratives and scenarios to support business or political proposals.
Data and System Dynamics .. 1+1=3 !
The emergence of data science had a strong synergistic effect on the development of system dynamics models.
Any computer simulation model needs a source of information or databases.
Forrester stated that system dynamics models must consider all the type of available information and databases (mental, written and numerical). Forrester considered the mental databases as the primary source of information and the most “voluminous”. [1]
Moreover, the massive growth in social and business data led to increased need to understand the behavior of those social or business systems.
Some domains may benefit more from the merge between data science and system dynamics like “crime fighting, infectious diseases, cybersecurity, national safety and security, financial stress testing, market assessment, asset management, and future-oriented technology assessment” (Erik Pruyt et al., August, 2014) [2].
System Dynamics * 3Vs = 6Vs
Some reviews stated that the 3V’s that define big data (volume, variety and velocity) can be extended to include other V’s (veracity, validity and volatility)[3]. System Dynamics models was a very successful methodology to illustrate and explain the issue.
Tools
Stella, Vensim and Powersim are the most famous modeling and simulation tools.
As we explained, Integrating data science and big data can be an “add-value” to the modeling and simulation process. This can be done through some tools like (PySD). PySD is a good example where system dynamics models and big data can be integrated.
To drive successful outcomes in healthcare, data science is increasingly integrating advanced tools like Generative AI in Healthcare and Healthcare Chatbot applications. These innovations allow healthcare providers to analyze vast datasets for predictive insights, streamline patient interactions, and enhance personalized care. As system dynamics continue to evolve, these technologies play a pivotal role in adapting to and addressing the needs of modern healthcare environments, showcasing the synergy between advanced data analytics and patient-centered solutions.
References
[1] Forrester J. Some basic concepts in system dynamics. Sloan Sch Manag … [Internet]. 2009; 1-17. Available from: https://www.cc.gatech.edu/classes/AY2018/cs8803cc_spring/research_papers/Forrester-SystemDynamics.pdf [2] Pruyt E. From Data-Poor to Data-Rich: System Dynamics in the Era of Big Data. 32nd Int Conf Syst Dyn Soc. 2014;(1):1–12. [3] Dave Anderson , The Value of Big Data – Defining It Once and For All,2013, http://www.builtinchicago.org/blog/value-big-data-defining-it-once-and-all.